Improve AI Basics - Ranking
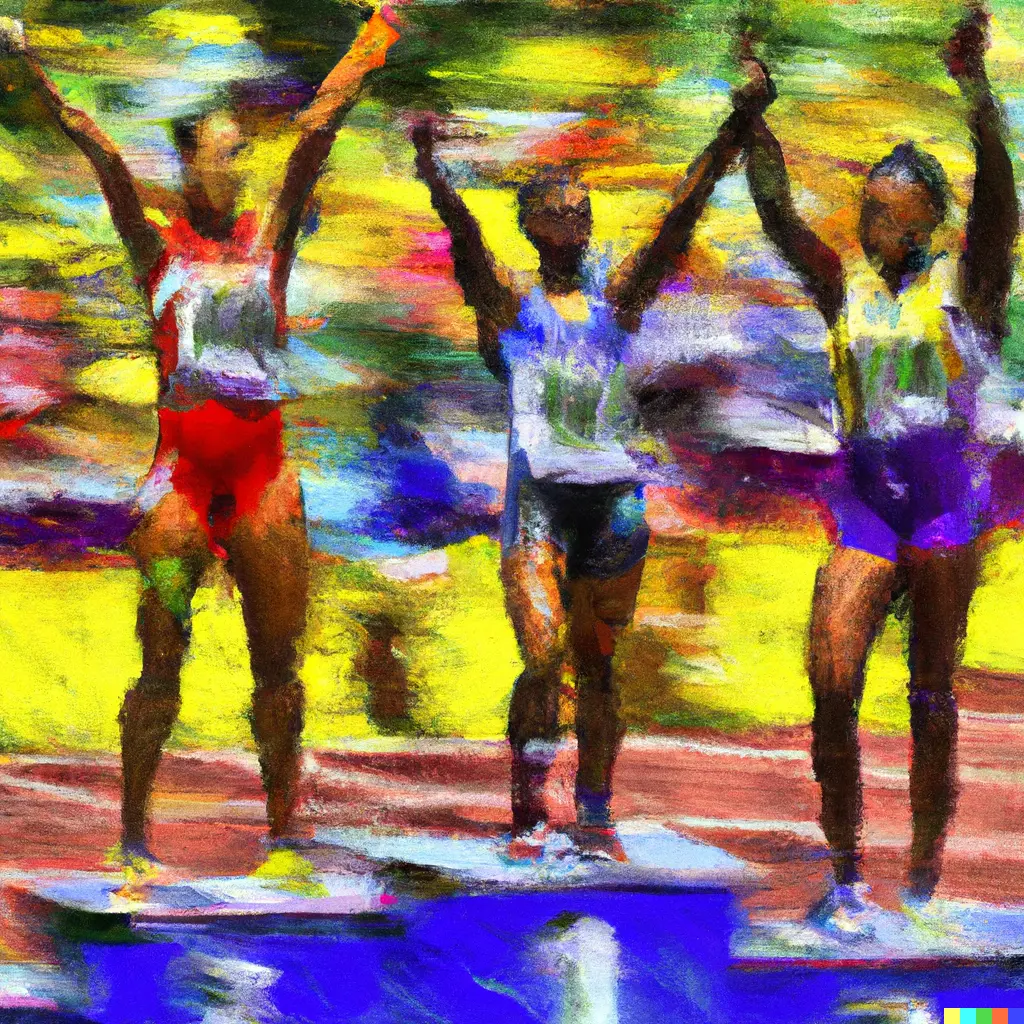
Ranking is a fundamental pattern in modern software development. From social media feeds, to product recommendations, to search engine results, it is often necessary to rank results before we display them.
rank()
Ranking can easily be performed with a single line of code:
wineRanking = sommelierModel.rank(wines)
DecisionModel.rank() is the fastest way to rank a large list of variants. It simply computes a score for each variant, then sorts the variants by their scores.
Just as with a normal decision, after the ranking is returned, rewards are assigned via addReward().
if (success) {
sommelierModel.addReward(1.0)
}
As with which(), the context is taken into consideration when making the ranking.
wineRanking = sommelierModel.given(entree).rank(wines)
On iOS and Android, contextual data such as operating system, country, language, time of day, and more is automatically included. Custom context can also be provided via given().
Ranking and Decisions
rank() is closely related to decisions made via which(), in fact, the following two statements are equivilent:
best = model.which("a", "b", "c")
and
best = model.rank(["a", "b", "c"])[0]
This equivilence means that a model trained on decisions from which() can also be used for rank() and vice versa.
“If you ain’t first you’re last.” - Ricky Bobby
Like which(), rank() only cares about the top position. The tradeoff for speed is that it doesn’t consider the relationships between the items themselves, and yet this simple pattern often performs quite well in practice.
Re-Ranking
Re-ranking is a powerful design pattern that allows seperation of concerns between content recommendation systems and optimizing the final display for the end user.
Rather than just competing for the top spot, re-ranking typically also considers the relationships between the items and their relative positions.
There are many different re-ranking algorithms, from listwise approaches with combinatorial complexity to pairwise approaches that essentially quicksort pairs of decisions. All of these can use the core foundation of which() and rank() to build higher level algorithms.
We will be writing more about re-ranking in the future, but in the mean time please contact [email protected] with any questions.
Recent Posts
Improve AI 8.0 - Contextual Multi-Armed Bandit Platform for Scoring, Ranking & Decisions
We’re thrilled to introduce Improve AI 8.0, a modern, free, production-ready contextual multi-armed bandit platform that quickly scores and ranks items using...
Simple Re-Ranking of SQL Queries with Machine Learning
In this tutorial, we will demonstrate how to use the Scorer and RewardTracker classes to update a ‘score’ column for a number of products in a ‘products’ tab...
Making Decisions with Ranker
In this tutorial, we’ll walk through how to use the Ranker class to make decisions, specifically choosing a discount to offer from a list of discounts. We’ll...
Optimize Any Python, Swift, or Java Object with Reinforcement Learning
Improve AI is a machine learning platform for making apps self-improving, meaning they optimize their own data structures and variables to improve revenue an...
Self Improving Apps: The Origin of a Radical Idea
I’ve been working on Improve AI for many years, but by 2017 the idea had begun to gel enough that I was ready to talk about it.
Improve AI - Easily Optimize Your App with Reinforcement Learning
Optimize and personalize your apps with fast AI decisions that get smarter over time. Decisions are made immediately, on-device, with no network latency. Th...
The NEW 20 lines of code that will beat A/B testing every time
In 2012, Steve Hanov wrote the popular and controversial blog post “20 lines of code that will beat A/B testing every time” that brought the previously acade...