Improve AI Basics - Scoring
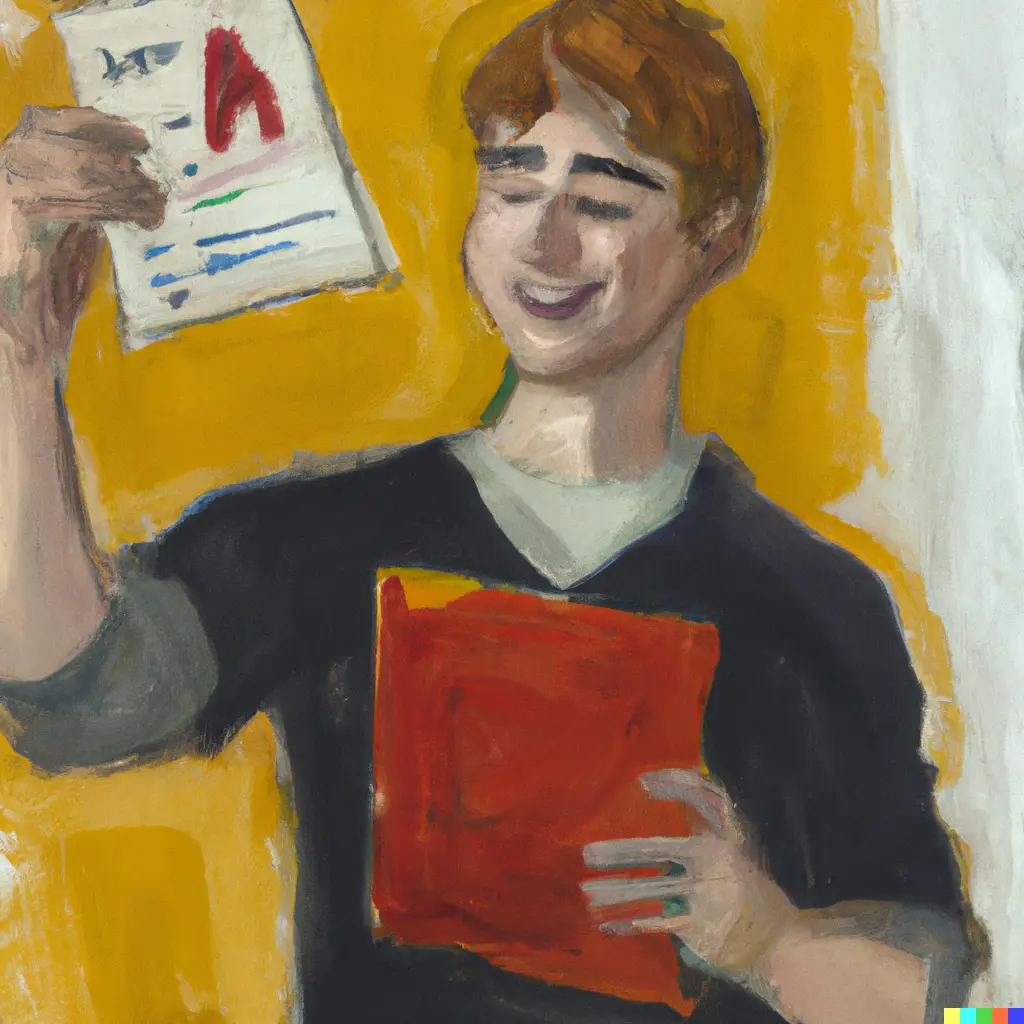
Scoring is another tool for optimization and recommendations that can reduce latency and decrease costs.
Scores are useful in a couple of ways. First, scores can be pre-computed and stored as a column in a database. This makes it easy to query items and sort them by their scores, saving precious milliseconds from doing a separate ranking step.
Scores are also useful for estimating statistics that can be input into further steps in a multi-stage recommendation system. For example scoring models could estimate click through rates, conversions, or any other metric that might be useful to a personalization or recommendation process. Since scoring is executed locally against the model, no network latency is incurred and additional systems for gathering statistics, such as Redis, don’t need to be deployed or managed.
Scoring is simple:
scores = sommelierModel.score(wines)
Scoring is useful for persisting scores in advance in a database and sorting queries by those scores.
score()
scores = DecisionModel["recommendations"].score(items)
Rewards are not tracked via the score interface. which(), rank(), or decide() must be used to train models for scoring.
Recent Posts
Improve AI 8.0 - Contextual Multi-Armed Bandit Platform for Scoring, Ranking & Decisions
We’re thrilled to introduce Improve AI 8.0, a modern, free, production-ready contextual multi-armed bandit platform that quickly scores and ranks items using...
Simple Re-Ranking of SQL Queries with Machine Learning
In this tutorial, we will demonstrate how to use the Scorer and RewardTracker classes to update a ‘score’ column for a number of products in a ‘products’ tab...
Making Decisions with Ranker
In this tutorial, we’ll walk through how to use the Ranker class to make decisions, specifically choosing a discount to offer from a list of discounts. We’ll...
Optimize Any Python, Swift, or Java Object with Reinforcement Learning
Improve AI is a machine learning platform for making apps self-improving, meaning they optimize their own data structures and variables to improve revenue an...
Self Improving Apps: The Origin of a Radical Idea
I’ve been working on Improve AI for many years, but by 2017 the idea had begun to gel enough that I was ready to talk about it.
Improve AI - Easily Optimize Your App with Reinforcement Learning
Optimize and personalize your apps with fast AI decisions that get smarter over time. Decisions are made immediately, on-device, with no network latency. Th...
The NEW 20 lines of code that will beat A/B testing every time
In 2012, Steve Hanov wrote the popular and controversial blog post “20 lines of code that will beat A/B testing every time” that brought the previously acade...